
Nature provides a wealth of bioactive compounds with a wide range of potential applications. However, only about 1% of these compounds have been identified.
LINNA is our Artificial Intelligence platform, which combines multiple predictive models with a primary goal: to uncover novel, untapped bioactive compounds hidden in nature and to identify potential applications for known compounds at a speed and precision beyond human capacity.
Learn more about LINNA®'s analytical capabilities.
In recent years, new and emerging learning methodologies call for algorithms to focus on non-obvious aspects. For instance, in language or image models, techniques like hiding a portion of the picture or sentence allow the model to continue making predictions even when the input is erroneous or missing, forcing it to focus on more subtle aspects that may otherwise go unnoticed. Reinforcement learning is used in the video game industry as well. In this type of learning, the algorithm explores strategies and receives positive or negative feedback based on their success or failure. It discovers strategies that a human would never be able to uncover by thoroughly evaluating the breadth of motions and plays.
LINNA employs several of these advanced learning methods to better understand the natural world. It can, for example, predict the intricate responses of protein interaction networks, as well as the regulatory mechanisms and the impact of minor disturbances on these very sensitive networks. Thanks to the vast amount of data, LINNA learns to draw conclusions based on metabolite properties or genomic context, rather than relying solely on the stricter and more descriptive methods employed by human scientists. Due to its capacity to draw conclusions, LINNA is able to analyze millions of candidates and compare them to multiple interactome regions of the model organism. It reveals less obvious interactions and responses that may be challenging for humans to grasp.
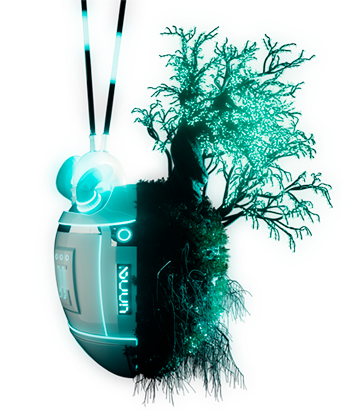
Natural Intelligence
We begin by tapping into Natural Intelligence, leveraging the wisdom accumulated over millennia of evolution. We meticulously study the intricacies of nature’s mechanisms, unveiling its hidden compounds and harnessing its richesthrough biotechnological processes such as pyrolysis, fermentation, and extraction.
Botany, Microbiology, and Microalgae provide an infinite number of potential compounds, which we utilise to develop productive solutions for the future.
SOLUTIONS FOR YOUR CHALLENGES
Why LINNA®?
LINNA was conceived to address the demands of the agricultural sector. However, given its objectives and immense potential, its application may grow beyond agriculture and into areas such as agribusiness, dermo-cosmetics, and the pharmaceutical industry.
LINNA, proposes to address all of these challenges by discovering new applications for the 1% of known bioactive compounds as well as searching for novel bioactive compounds among the unknown 99%.
Learn more about LINNA®'s fundamental purpose.
With its expansive potential, LINNA is empowered to explore synergies within bioactive compounds, unveil novel mechanisms of action, predict potential toxicity, and uncover non-evident realities—those intricate patterns in nature that ordinary human observation fails to reveal.
Our unwavering efforts, driven by ambition, propel us forward. Our goal is to comprehensively analyze between 3 and 5 million natural compounds by 2030. Our goal is to obtain innovative solutions, ranging from a novel herbicide to replace the use of glyphosate to identifying viable alternatives for the chemical preservatives used within the food industry.
How Does LINNA® work?
In order to predict the activity of natural compounds and, consequently, prescribe new ones to address a specific need, LINNA® comprises multiple prediction models that are seamlessly integrated with one another.
To achieve this, we employ a variety of extraction and transformation techniques to access the complete chemical spectrum found within various natural raw materials. Through this approach, we can pinpoint more than 10 complex candidates for each analyzed raw material. Each of these candidates, consisting of hundreds of unidentified compounds, triggers a specific response when administered to an organism. By understanding these responses, through diverse methodologies based in systems biology and Big Data, LINNA® has the capacity to predict an organism’s behavior concerning various candidates, all without the need for conventional testing.
1. DataDiscovery
2. DataShine
3. LINNABrain
4. LINNALearn
1. DataDiscovery.
Accesses internal and external data.
- We analyze 3 to 5 million natural compounds that originate from our own candidate amplification and generation node. This collaborative approach with science makes us significantly more competitive. Would you like to know how we do it?
- Over 500 TB of transcriptome data
- Over 130 million images taken with RGB, PAM sensors and hyperspectral cameras.
All of this information, together with the external data, will allow us to discover previously unknown compounds and mechanisms of action while minimizing the requirement for experimental testing by up to 95%.
Internal Data
A proprietary universe of data that makes our platform unique
To achieve high efficacy, we use data generated internally in our value-search ecosystem, such as molecular profiles, cause-effect relationships between natural molecules and mechanisms of action against a target, and the knowhow on relationships and synergies between natural molecules that we have been developing for 15 years
External Data
External data sources that help us provide value and identify relevant patterns
For instance, we use data on historical temperature data and its correlation with crop performance, crop-specific farm monitoring using IoT devices, external databases for metabarcoding, and metagenomics to explore chemical compound structure-activity relationships.
2. DataShine.
It highlights data through a range of machine learning models, including regression, dimension reduction, and unsupervised clustering, to execute diverse tasks. These tasks encompass everything from exploring a candidate’s chemical space to characterizing the transcriptome response to its application. Additionally, it employs deep learning models such as graph neural networks and encoder-decoder models to improve biological activity predictions.
All these models are integrated to create three modules, transforming LINNA into an unrivaled, potent, and adaptable tool. Utilizing these modules has enabled us to uncover 30 candidates possessing formidable herbicidal potential—discoveries that would have eluded us relying solely on human reasoning.
LINNA Modules
LINNA comprises three core modules – expression, dimensionality reduction, and phenotype – seamlessly integrating multiple models into a unified pipeline which aims not only to forecast a phenotype from a complex candidate but also to propose novel bioactive compounds necessary for attaining a desired phenotype or biological activity:
1. Expression
LINNA®’s “expression” module predicts changes in expression in an organism of interest based on candidate information acquired through high-resolution spectrometry techniques. All of this information is cross-referenced with the LINNA® databases to identify the known compounds for which a fingerprint is calculated. This module also includes neural networks for estimating the structure and fingerprint of unknown compounds.
The candidate’s data is utilized to predict the expression profile that the candidate as a whole and each of its constituent compounds would generate in the model organism.
2. Dimensional Reduction
Dimensional reduction obtained by LINNA® module 2 following the prediction of over 5000 differentially expressed genes. This representation highlights the various modified biological processes, their constituent genes, associated gene ontology, and the interactions between the proteins encoded by these genes.
3. Phenotype
The third module predicts a wide variety of phenotypic characteristics using the biological processes affected by the research candidate as input. The mathematical models included in this module can not only predict these parameters, but also assign the relative importance that each of them will have in the final phenotype.
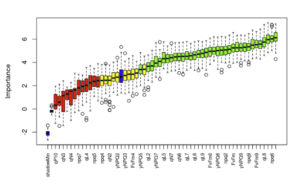
Relative importance allocation for phenotypic characteristics predicted by LINNA® module 3.
All of these parameters, together with their relative importance, allow us to categorize the research candidate (or any of its bioactive compounds) not only by biological activity or predicted phenotype, but also by mechanism of action within each biological activity. This grouping is compared to the prior module’s expression data, allowing for complex correlations between transcriptome and phenotypic response in a reduced dimensional space.
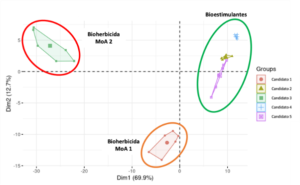
Grouping of five candidates examined in biological activities and mechanisms of action by LINNA® module 3 based on phenotypic parameter predictions and relative importance.
3. LINNABrain.
Our rationale is based on business intelligence, commercial strategies, the real specific needs of the industry, market demand, and other factors, all while providing a 360-degree solution that addresses a real need in the industry.
4. LINNALearn.
It gains knowledge from LINNABrain’s decisions and improves results by increasing its prescription capacity.
If you’ve really come this far…
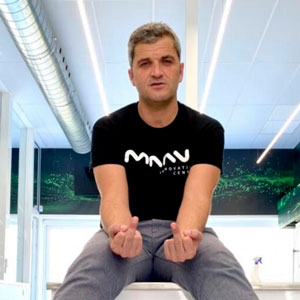
You are obsessed with knowledge
You are from the competition and surely…
You have been offended so do not follow our CEO on Instagram
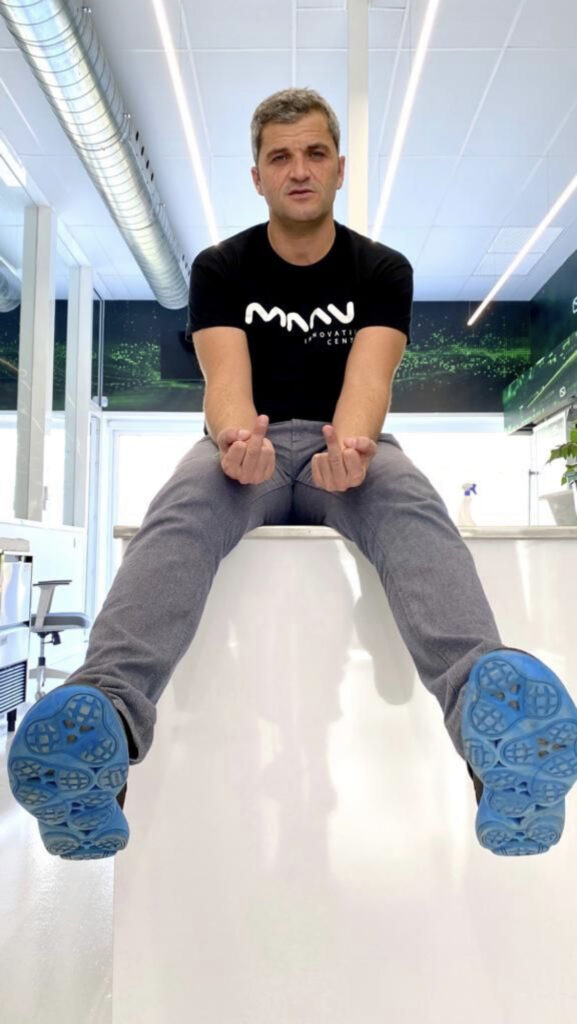
LINNA®’s transversality
LINNA was created to address agricultural challenges by developing bioherbicides, biostimulants, probiotics, biofungicides, bioinsecticides, and seed treatment; however, its immense potential makes it a transversal platform capable of addressing challenges in animal production, fish farming, and the cosmetic and pharmaceutical industries.
Anticipating the future
Our target for 2030
is to analyze 3-5 million natural compounds with a prediction accuracy surpassing 95%.
To benefit from non-evident reality, which is defined as AI’s capacity to find hidden relationships that are undetectable through mere observation.
To manage and analyze a huge amount of data thanks to our extensive experience with natural compounds acquired over the last 15 years.
To explore transversality, as the laws of biology are universally applicable to all organisms.
To study the 99% of unknown natural compounds.
To find new mechanisms of action associated with natural molecules.
To predict possible toxicities in our products.
To look for synergies between natural molecules.
To find new uses for the 1% of already known natural compounds.
They have seen the future
Kimon Theophanopoulus
CEO & Founder Teofert
Alexandre Macedo
Vice President of Biostimulants at Yara Europe
Ernesto Baltodano
General Manager at CISA Agro
Natural bioactive compounds
Bioactive compounds are naturally occurring chemical substances with specific biological activities which have traditionally been used in the food, dermocosmetics, and pharmaceutical industries.
Bioactive compounds are produced by various organisms, including plants, fungi, bacteria, and microalgae.
Among these, plants stand out as true biofactories of phytochemicals, which are bioactive molecules produced by plants themselves. Plants naturally synthesize these phytochemicals through their secondary metabolism, which involves a series of chemical reactions leading to the formation of a variety of compounds. While these molecules are not essential for the plant’s survival, they play a crucial role in maintaining its overall health; for instance, they aid in environmental adaptation and pathogen defense, as well as improving pollination through the production of pigments.
When it comes to microorganisms and microalgae, our understanding encompasses merely 1% of their biodiversity. With LINNA®, we can find novel applications for compounds derived from these microorganisms and microalgae, as well as identify the remaining 99% of microorganisms and their compounds. Without a question, there is still a great deal of untapped potential.
Among these phytochemicals, notable examples include:
- Salicylic acid, extracted from willow bark, serves as an effective treatment for acne in dermocosmetics due to its anti-inflammatory and antibacterial properties.
- Quinine, derived from the bark of Cinchona trees, is used as an antimalarial agent, a component in anti-hair loss shampoos, and as a flavor enhancer in tonic water.
- Morphine, an exceptionally potent pain-relieving compound, is derived from the opium poppy (Papaver somniferum).
- Artemisinin, sourced from the herb Artemisia annua, plays a key role in an innovative malaria treatment; its discovery earned Tu Youyou the Nobel Prize in Medicine in 2015.
Artificial Intelligence
Artificial intelligence allows us to analyze millions of data points and uncover hidden patterns. That is why LINNA® employs algorithms and prediction models that include machine learning and deep learning approaches.
LINNA® combines regression models, dimensional reduction, and unsupervised clustering to carry out tasks such as exploring a candidate’s chemical space or characterizing an organism’s transcriptomic response upon the application of the said candidate.
These machine learning models are supplemented by graph neural networks and encoder-decoder models, improving predictive capabilities and establishing LINNA® as a powerful and versatile tool.
A groundbreaking approach that revolutionizes the way scientific research is conducted.
Future
Accelerating scientific research processes
Know-how
We minimize failure and retain knowledge.
To achieve this, LINNA® leverages the Natural Intelligence gathered over 15 years of research into the processes necessary for accessing the entire chemical spectrum of natural raw materials. This entails understanding how bioactive compounds behave and how they interact with one another to create synergies. Our Natural Intelligence is powered by Artificial Intelligence, making LINNA® a game-changing tool. Botany, Microbiology, and Microalgae comprise a wealth of unknown compounds that could potentially be used to develop productive solutions for the future.
Analyzed natural compounds
By 2030, LINNA® will complete the analysis of 3 to 5 million natural compounds, generate more than 500 TB of transcriptome data, and amass over 130 million images using RGB, PAM sensors, and hyperspectral cameras. Cross-referencing this vast amount of data with our databases will enable us to identify novel compounds and previously unidentified mechanisms of action. This approach will considerably reduce the need for experimental trials by up to 95%.
Our databases, which were generated to provide LINNA® with the information it needs to expand its predictive capabilities, include
450.000+
natural molecules
270+ million
synthetic molecules
200+ million
proteins and their corresponding isoforms have been analyzed to date.
2000+
genomes and proteomes from various plant, fungal, bacterial, and animal species.
2+ billion
protein-protein interaction networks.
500+
shrared metabolic pathways.
Learn more about how LINNA works.
At its core, LINNA® is founded on systems biology, which is an in-depth study of a biological system with the goal of gaining a greater understanding of it and, therefore, facilitating the possibility of predicting its behavior in the future. LINNA® does this by integrating mathematical models with biological processes, allowing us to predict traits and behaviors of living organisms that may elude direct observation.
Considering the intricate complexity of living organisms, attaining a profound understanding of them proves to be a challenging task. In order to unravel this intricacy, LINNA® draws upon extensive biological data, commonly referred to as omics data, as the fundamental building blocks for predictions generated by its mathematical models.
Learn more on the data used to train LINNA®
LINNA’s backbone comprises the natural candidates subjected to different challenges, serving as a primary data source that nourishes its predictive models. Hence, characterizing the chemical profile of these candidates and identifying their constituent compounds becomes indispensable. To that end, all candidates are thoroughly studied using various mass spectrometry techniques.
The application of these candidates, on the other hand, causes a succession of changes in the tested individual, first at the molecular level and subsequently at the phenotypic level. Hence, we collect transcriptome data through massive sequencing and phenomic data via robotic phenotyping cameras for each organism exposed to a particular candidate. These omics data are analyzed using a variety of bioinformatics approaches to eventually fuel the prediction models, which are then generated and smoothly integrated into LINNA. As a result, LINNA has the capacity to correlate the activity of individual compounds within a complex candidate with its associated expression profile and then further connect all of these factors to specific phenotypic modifications.
Chemical profile
Chemical profiling involves a thorough molecular-level characterization of a candidate. To accomplish this, both the volatile profile is examined using gas chromatography-mass spectrometry (GC-MS), and the remaining compounds within the candidate are scrutinized via liquid chromatography-quadrupole-time-of-flight mass spectrometry (Q-TOF). This high-resolution mass spectrometry equipment enables us to detect a vast array of compounds within a candidate. The detection and subsequent identification of compounds feed a database internally used by LINNA, which uses its integration with other databases of already-existing natural compounds to provide suggestions for new compounds.
Combining these mass spectrometry techniques allows us to fully understand a candidate’s composition, which can be linked to an expression profile, transcriptome, or determined phenotype, using LINNA’s integrated prediction models.
Phenotyping
The phenotype encompasses an organism’s outward appearance and includes all observable and measurable traits that can be evaluated without causing harm to the subject under study. This encompasses dimensions, colors, and other properties that provide structural information. Furthermore, the phenotype includes the acquisition of physiological or biochemical features through non-invasive techniques.
LINNA employs phenomics data collected via a robotic phenotyping platform that integrates RGB, PAM sensors, and hyperspectral cameras. This platform allows us to get a comprehensive overview of all the phenotypic traits exhibited by an organism in response to the application of a candidate. In this manner, we can acquire data about protein content, water levels, and photosynthesis mechanisms in a plant treated with a particular candidate. This information is indispensable for LINNA to effectively decipher intricate biological systems, such as those found in plants.
Massive sequencing
Massive sequencing refers to the collective use of nucleic acid sequencing techniques carried out in tandem and on a large scale.
LINNA’s predictive models are fed with vast amounts of sequencing data, specifically transcriptomics data. This technique enables the assessment of the presence and quantity of all RNA molecules within a biological sample at a specific moment.
Bioinformatics
Bioinformatics is becoming increasingly important in the collection of massive biological datasets, such as the transcriptomic and phenomic data that power LINNA. To prevent the introduction of human biases that could lead to undesired deviations in predictions, we have meticulously designed workflows dedicated to the automated analysis and organization of the transcriptomic and phenomic data fueling the integrated predictive models within LINNA.
LINNA®’s transversality
Transversality is one of LINNA’s distinguishing features. Not only can it predict compound activity and propose new compounds for agricultural application, but it can do the same for any challenge we propose.
To do this, our candidates are tested on a series of carefully selected model species in order to collect molecular information on how these organisms respond to the tested candidate.
LINNA® can predict the molecular alterations that would occur in other organisms if we tested these candidates on them using this molecular information and a mathematical model designed specifically for this purpose.
Learn more about the predictive models applied to LINNA®‘s transversality.
Learn more about the predictive models applied to LINNA®’s transversality
Model organisms are organisms that are commonly utilized by researchers for studying specific biological processes.
Jacques-Lucien Monod famously stated, ‘Whatever is true for Escherichia coli must also hold true for elephants.’ Based on his insights into gene expression regulation in bacteria, which could be extended to all life forms, Jacques-Lucien Monod received a Nobel Prize in Medicine in 1965. His ideas also sparked the creation of LINNA’s predictive mathematical model for transversality.
By utilizing transcriptomic data from the model organism’s response to the tested candidate, annotating orthologs between the model organism and the target organism, and considering the genomic context of both organisms, we’ve developed and incorporated into LINNA a mathematical model. This model can predict the differential expression changes that a candidate will induce in another organism, eliminating the necessity for redundant testing.